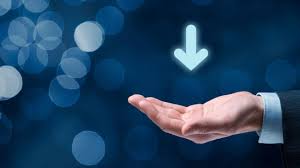
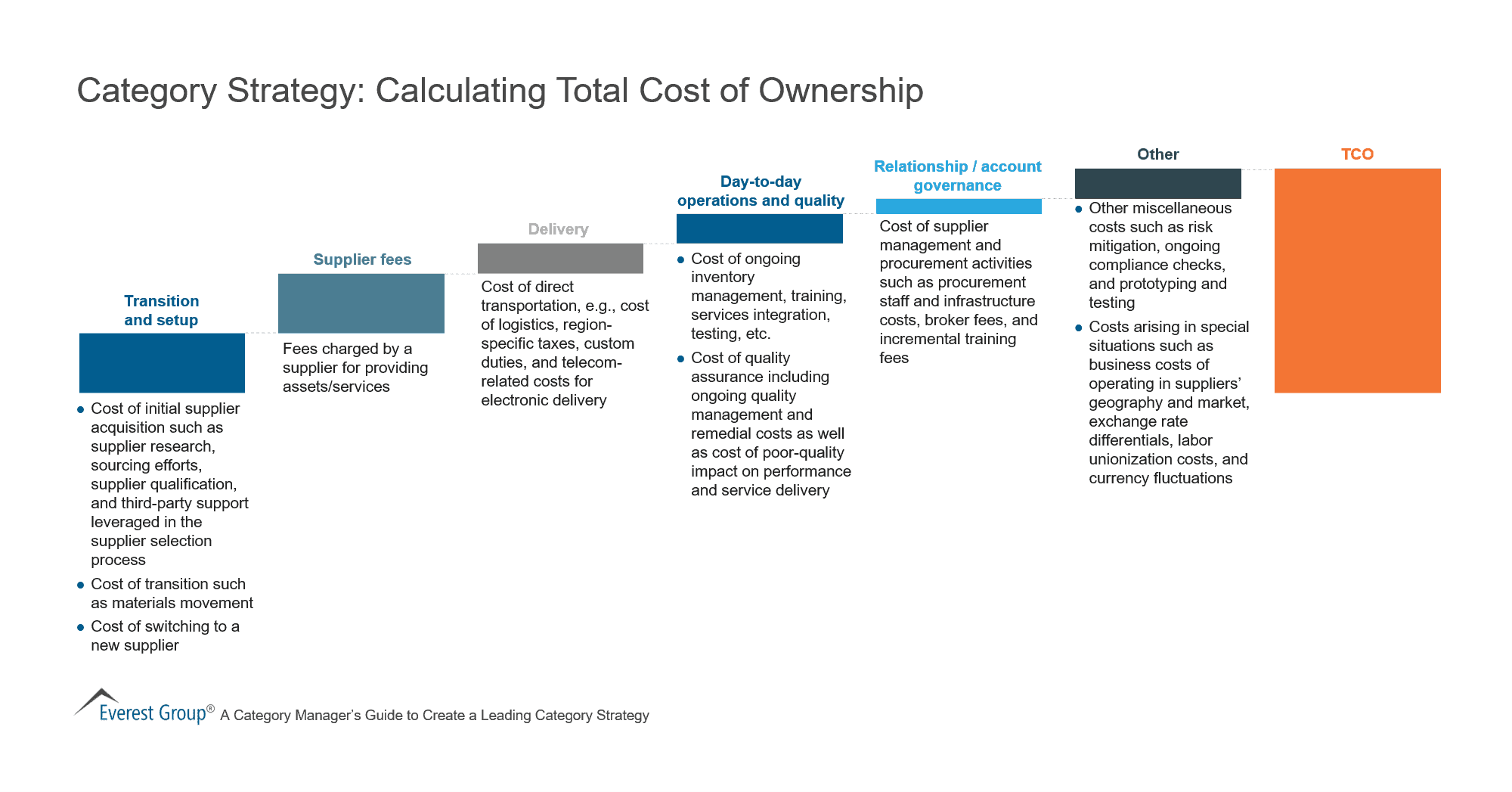
This context includes the arm and any other object that coherently moves along with the hand. In this paper, we propose an unsupervised method to automatically learn the context in which a hand is embedded. However, robust and real-time hand tracking in unconstrained environments remains a challenging task due to the high number of degrees of freedom and the non-rigid character of the human hand. Hand tracking in video is an increasingly popular research field due to the rise of novel human-computer interaction methods. Besides, extensive experiments on five benchmarks validate the effectiveness of SSR.

Finally, the proposed SSR is used to equip three popular spatially-regularized CF trackers to significantly boost their tracking accuracy, while achieving much faster online tracking speed. Moreover, by adding a special state, representing not-updating filters, in the MDP, we can learn when to skip unnecessary or erroneous filter updating, thus accelerating the online tracking. We then formulate the online selection of these weight maps as a decision making problem by a Markov Decision Process (MDP), where the learning of weight map selection is equivalent to policy learning of the MDP that is solved by a reinforcement learning strategy. Specifically, rather than simply relying on foreground information, we extend the objective function of CF tracking scheme to learn the target-context-regularized filters using target-context- driven weight maps. It can achieve not only higher accuracy and robustness, but also higher speed compared with spatially-regularized CF trackers. In this paper, we propose selective spatial regularization (SSR) for CF-tracking scheme. Nevertheless, SR highly increases the optimization complexity of CF and its target-driven nature makes spatially-regularized CF trackers may easily lose the occluded targets or the targets surrounded by other similar objects. Spatial regularization (SR) is known as an effective tool to alleviate the boundary effect of correlation filter (CF), a successful visual object tracking scheme, from which a number of state-of-the-art visual object trackers can be stemmed.

We also conduct comparison with a number of state-of-the-art trackers and demonstrate the competitiveness of the proposed method. By applying our SAT on several tracking problems, we show that location correction based on structural constraints is a key technique to improve prediction in moderately crowded scenes, even if only a small part of the target is visible. Finally, the location prediction is corrected by exploiting the keypoint structural model with a voting-based method.

Second, the target location is estimated in the reduced search space using deterministic keypoint matching. First, the search space is reduced to the most likely image regions with a probabilistic approach. With this multi-feature appearance model, our Structure-Aware Tracker (SAT) estimates accurately the target location using three main steps. The appearance model also incorporates the spatial layout of the keypoints, encoding the object structure learned during tracking. To represent the target, we use a new model combining color distribution with keypoints. This paper introduces a novel keypoint-based method for visual object tracking.
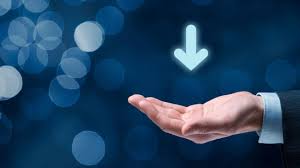